O1 is a new OpenAI model focused on reasoning and solving complex tasks, with enhanced capabilities in coding when integrated with the Devin coding agent. It aims to push the boundaries of artificial intelligence, making it smarter, more accurate, and better at solving complex tasks, including notable advancements in areas like mathematics and science. For example, in math benchmarks, O1 achieves an impressive 94.8% in comparison to GPT-4O’s 60.3%, and in physics, O1 scores 92.8% compared to GPT-4O’s 59.5%. In comparison to GPT-4O, the O1 model demonstrates significant improvements in various benchmarks, such as natural language understanding and task performance. For instance, O1 achieves 73.2% on MathVista compared to GPT-4O’s 63.8%, and on another benchmark (MMLU), O1 outperforms GPT-4O by reaching 92.3%, while GPT-4O scored 88.0%.
A key advancement in O1 is that the models are trained to spend more time thinking through problems before they respond. Through training, they learn to refine their thinking process, try different strategies, and recognize their mistakes. This approach enables O1 to provide more accurate and well-reasoned responses, especially in complex tasks. Key features of O1 include significant improvements in model architecture and training methodologies, enhancing performance and contextual understanding. In the realm of natural language tasks, for example, O1 achieves a performance of 83.3% in AP Calculus, while GPT-4O stands at 71.3%, highlighting O1’s ability to provide more nuanced and context-aware interactions.
OpenAI’s O1 models have demonstrated superior performance in coding environments, as evaluated by Cognition AI’s SWE-bench. Devin, utilizing O1-preview, achieved a 51.8% success rate in complex coding tasks compared to GPT-4O’s 25.9%, and the production version of O1 scored an impressive 74.2%. These results highlight O1’s ability to “think” through problems and correct mistakes, an essential skill in debugging and real-world software setups. O1’s advancements in multi-step planning and autonomous feedback allow it to handle tasks like configuring software environments, where previous models struggled.
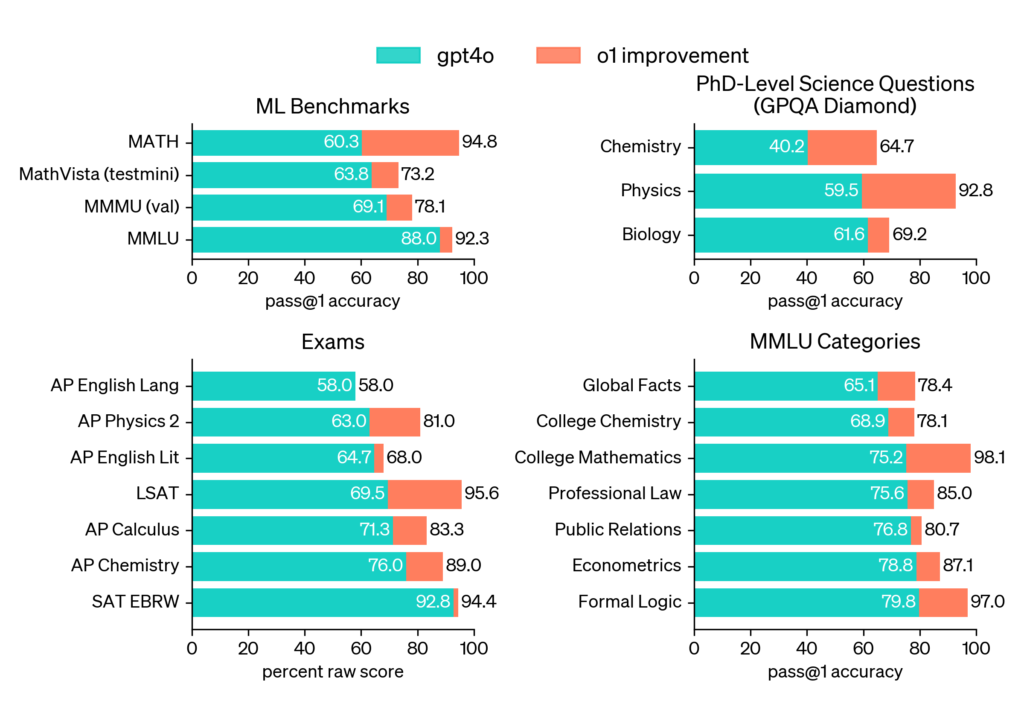